How Ai is aiding in fraud detection & Prevention
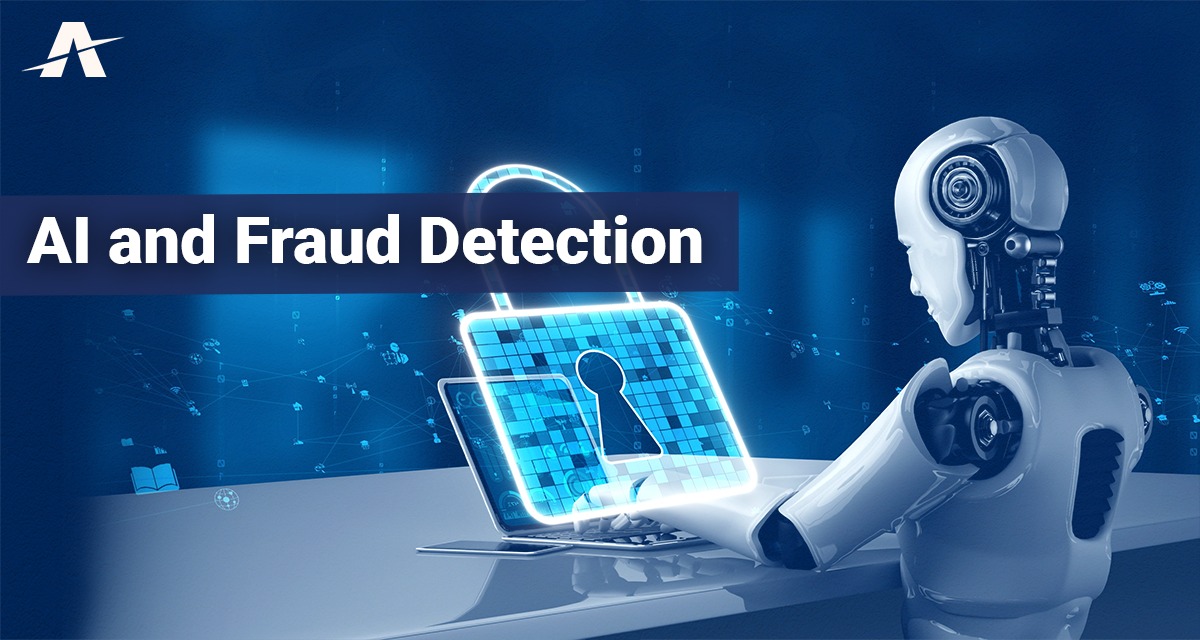
What is fraud detection and prevention?
Fraud detection and prevention are the processes and techniques that are utilized for identifying, analyzing and preventing fraudulent activities across various sectors such as finance, e-commerce, telecommunications and healthcare. Fundamental goal being protecting individuals and organizations from financial losses, security breaches and reputational damage caused by fraudulent activities.
The traditional methods and approaches for fraud detection are highly valuable and forms the foundation of the prevention and fraud detection AI Technology and is being deployed in various industries worldwide which we shall be covering in this artist including fraud detection by ai in preventive healthcare.
What is ai detection in preventing fraud?
AI algorithms are implemented for preventing fraud by employing various techniques and strategies for successfully detecting and mitigating fraudulent activities in real-time.
Let’s see how AI fraud detection prevention is done:
1. Anomaly Detection :
AI algorithms systematically analyze historical transaction data and user behaviour for establishing normal patterns. When deviations or anomalies are detected for example, unusually large transactions, atypical user behaviour or some irregular access patterns, AI system flags them for further investigation as potential fraudulent activity.
2. Pattern Recognition :
AI models meticulously utilize machine learning techniques for identifying patterns and correlations in data that may indicate fraudulent behaviour. By continuously monitoring and analyzing transaction data and user interactions, AI systems get trained in recognizing common fraud patterns and proactively prevent fraudulent activities before they can even occur.
3. Predictive Analytics :
AI implements predictive analytics for forecasting potential fraud risks that are based on historical trends and patterns. AI systems are programmed to take preventive measures by blocking suspicious activities or by alerting fraud prevention teams for further action when identifying these high-risk transactions or users in advance.
4. Behavioural Biometrics :
AI-based systems are designed to use behavioural biometrics like the keystroke dynamics, mouse movements and voice patterns for the process of authenticating users, detecting unauthorized access and also for account takeover attempts. AI can identify anomalies and flag them as potential fraud by continuous monitoring of user behaviour.
5. Machine Learning Models:
AI employs machine learning models to learn from new data and subsequently adapt to evolving fraud patterns over time. AI systems can keep themselves ahead of emerging fraud threats and prevent future incidents by continuously updating their detection algorithms which are based on feedback from the already detected fraud cases.
6. Real-time Monitoring :
AI-based fraud prevention systems are designed to monitor transactions and activities in real-time, this allows for immediate detection and response to any fraudulent behaviour just as it occurs. Implemented AI models can therefore quickly identify and block fraudulent transactions before they are completed just by analyzing data streams in real-time.
7. Automated Decision Making :
Deployed AI models are programmed in enabling automated decision-making processes which can instantly approve legitimate transactions and flag suspicious ones for reviewing later. AI systems are capable of reducing response times and minimizing the risk of human error just by automating routine fraud detection tasks.
AI thus plays a significant and crucial role in preventing fraud by continuously analyzing data and detecting anomalies and patterns which are indicative of fraudulent activity and taking proactive measures to stop fraudsters in their tracks.
The AI strategies utilized for fraud detection and prevention
AI employs different strategies for fraud detection and prevention, utilizing algorithms and techniques for analyzing data, detecting patterns and also for identifying anomalies that are indicative of fraudulent activity.
Let’s see some of the key AI strategies utilized for fraud detection and prevention:
Machine Learning :
Machine learning algorithms like supervised learning, unsupervised learning and semi-supervised learning are trained on input historical data for recognizing differently similar patterns and relationships between features that are associated with fraudulent and legitimate transactions.
Anomaly Detection :
Anomaly detection techniques are utilized for identifying deviations from normal behavioural patterns trained in data which helps indicate fraudulent activity. AI models are then able to detect anomalies in transaction amounts, user behaviour, access patterns and any other variables.
Predictive Analytics :
Predictive analytics utilizes the historical input data and machine learning models for forecasting any potential fraud risks and preventively identify all high-risk transactions or users before any fraudulent activities occur.
Behavioural Biometrics :
Behavioural biometrics strategy analyzes unique behavioural characteristics of users like the keystroke dynamics, mouse movements and also the voice patterns for authenticating users and detecting unauthorized access or any account takeover attempts.
Entity Link Analysis :
Entity link analysis strategy is utilized for examining relationships and connections between entities like individuals, accounts, devices and also in transactions for identifying suspicious links or networks that are indicative of fraudulent behaviour.
Natural Language Processing (NLP) :
NLP techniques is utilized for analyzing text data, like emails, chat logs and social media posts for identifying fraudulent communications, phishing attempts or any form of fraudulent advertising content.
Deep Learning :
Deep learning neural networks like convolutional neural networks (CNNs) and recurrent neural networks (RNNs) are utilized for analyzing complex and high-dimensional data like images, videos and even sequences for fraud detection and prevention.
Real-time Monitoring :
AI systems are utilized for monitoring transactions and activities in real-time, this allows immediate detection and response to any form of fraudulent behaviour just as it occurs thereby minimizing the impact of fraudulent activities.
Automated Decision Making :
This strategy allows AI in enabling automated decision-making processes which can instantly approve legitimate transactions and also flag suspicious ones for further review or intervention thereby reducing response times and also minimizing human error.
There's another strategy which is utilized for fraud detection and prevention:Ensemble Learning :
Ensemble learning strategy is a combination of multiple machine learning models utilized for improving the overall prediction accuracy and robustness. Different models like the decision trees, support vector machines and neural networks are thus trained independently on the same dataset and then subsequently their predictions are aggregated for making a final decision. Ensemble methods like random forests, gradient boosting and stacking further enhance fraud detection by capturing diverse perspectives and compensating for the weaknesses of individual models.
This strategy is especially effective in fraud detection as it is capable of reducing overfitting, increasing generalization performance and providing more reliable predictions by combining the strengths of multiple models. This particular approach helps organizations build more robust and adaptive fraud detection systems that are capable of accurately identifying and preventing fraudulent activities across various scenarios and data types.
The successful usecases and the industries benefitting .
Let’s see some specific use cases where AI-based fraud detection has been effectively employed:
Payment Fraud Detection :
AI algorithms are programmed for analyzing transaction data, user behaviour and historical patterns for detecting fraudulent payment transactions, unauthorized credit card use, account takeover or even identity theft.
- Industry: Banking and Financial services
Insurance Fraud Detection :
AI models are designed to analyze claims data and the policyholder information, they can even identify suspicious claims in historical fraud cases like exaggerated injuries and falsified accidents or even fraudulent insurance applications.
- Industry: Insurance
Banking and Financial Fraud Detection :
AI systems are deployed for monitoring banking transactions, account activities and customer interactions for detecting the different types of financial fraud, including account fraud, loan fraud, money laundering and even insider trading.
- Industry: Banking and Financial services
E-commerce Fraud Detection :
AI algorithms are proficient in analyzing online shopping behaviour, transaction histories and device information for detecting fraudulent activities like account takeover, payment fraud, fake reviews and even fraudulent returns.
- Industry: E-commerce
Healthcare Fraud Detection :
AI-based systems can efficiently analyze medical claims data, patient records and billing information for identifying fraudulent healthcare practices like billing for unnecessary procedures, up coding and even prescription fraud.
- Industry: Healthcare
Cybersecurity Threat Detection :
AI implemented models effectively analyze network traffic, system logs and user behaviour for detecting cyber threats like malware infections, phishing attacks, insider threats and also anomalous access patterns.
- Industry : Information Technology/ Cybersecurity
Telecom Fraud Detection :
AI algorithms are capable of analyzing call detail records, subscriber data and usage patterns to detect any kind of telecom fraud like subscription fraud, premium rate service fraud and even SIM card cloning.
- Industry: Telecommunications
Government Fraud Detection :
AI-based systems are equipped in analyzing government transactions like the benefit claims and even the taxpayer data to detect fraud in areas like tax evasion, social welfare fraud, unemployment insurance fraud and even the public procurement fraud.
- Industry : Governments/ Public Sector
Retail Fraud Detection :
AI algorithms systematically analyze retail transaction data, customer behaviour and inventory records for detecting fraudulent activities like return fraud, discount abuse, gift card fraud or employee theft.
- Industry : Retail
Travel and Hospitality Fraud Detection :
AI systems are built for effectively monitoring booking transactions, loyalty program activities and traveller behaviour to identify fraudulent activities such as credit card fraud, booking scams, identity theft or even the fraudulent claims for compensation.
- Industry: Travel and Hospitality
Supply Chain Fraud Detection :
AI-based systems are designed for systematically analyzing supply chain data, procurement records and vendor information for detecting fraud and corruption like invoice fraud, bid rigging, product substitution or collusion among suppliers.
- Industry : Supply Chain/ Logistics
Online Advertising Fraud Detection :
AI algorithms thoroughly analyze digital advertising data, website traffic and user engagement metrics for detecting fraudulent activities such as click fraud, impression fraud, ad stacking and even the bot traffic.
- Industry : Advertising/ Digital Marketing
Insurance Claim Fraud Detection:
AI models are deployed for analyzing insurance claims data, medical records and historical patterns for identifying suspicious claims like staged accidents, medical billing fraud, fraudulent property damage claims or even fake injury claims.
- Industry: Insurance
Pharmaceutical Fraud Detection :
AI-based systems precisely analyze pharmaceutical sales data, prescription records and also the regulatory compliance information for detecting fraudulent activities such as counterfeit drugs, illegal drug diversion or off-label marketing.
- Industry: Pharmaceuticals / Healthcare
Energy and Utilities Fraud Detection :
AI algorithms accurately analyze energy consumption data, meter readings and billing information for detecting fraud in areas like meter tampering, energy theft, billing fraud or unauthorized access to utility services.
- Industry: Energy and Utilities
These are only a few of the use cases demonstrating the versatility and effectiveness of AI-based fraud detection across a wide range of industries and domains, helping them in proactively identifying and mitigating fraud risks and thereby protecting their assets, reputation and stakeholders.
A notable real-time example
One notable example of AI being deployed by law enforcement for fraud detection and prevention is the collaborative partnership between the UK's National Crime Agency (NCA) and IBM’s AI to combat financial crime was announced in October 2018.
Context :
Financial fraud and cybercrime worldwide are significant challenges for law enforcement agencies. The NCA as a part of it strategy for enhancing its capabilities in detecting and preventing such crimes by leveraging advanced technologies build this partnership.
Data Integration :
The NCA worked relentlessly with IBM to integrate all data from various sources, including financial transactions, social media and public records. This comprehensive data integration from all the sources enabled a more comprehensive and holistic view of any potential fraud activities.
Machine Learning Algorithms :
IBM from its end provided machine advanced learning algorithms for analyzing this data and identifying patterns to detect anomalies that were indicative of fraudulent behaviour. The algorithms were capable of sifting through those massive datasets much faster and more accurately than the traditional manual methods.
Real-time Monitoring :
The AI system made way for the NCA to continuously be monitoring financial transactions and other relevant activities in real-time. This became the enabling factor for the agency to receive immediate alerts just as any suspicious activities were detected.
Link Analysis and Visualization :
The AI tools which also included advanced link analysis and visualization capabilities helped investigators in mapping out connections between different entities that were involved in fraud networks thereby making it easier to identify key players and in understanding the structure of criminal organizations.
Predictive Analytics :
Predictive analytics were employed in forecasting any future fraud risks and for identifying the potential hotspots for a financial crime. This proactive approach proved pivotal as it allowed the NCA in allocating resources more effectively and preventing crimes before they occurred.
Implementation:
Outcomes :
1. Enhanced Detection :
The deployed AI system proved useful and significantly improved the NCA's abilities in detecting complex financial fraud schemes that might have otherwise gone unnoticed with traditional methods.
2. Efficient Investigations :
By automating the process of data analysis and by providing actionable insights, the AI tools are enabling investigators to focus on the most promising leads and in building stronger cases against fraudsters.
3. Prevention :
Real-time monitoring and predictive analytics the core features of AI technology is helping prevent fraud by enabling timely intervention and in preventing criminal activities.
This collaborative partnership between the NCA and IBM illustrates how AI can be effectively utilized by law enforcement agencies for enhancing their capabilities in fraud detection and prevention thereby leading to more efficient and successful investigations.
The challenges and risks involved with AI technologies in fraud detection and prevention systems:
While AI-based fraud detection and prevention systems offer significant benefits, there are also several risks and challenges that are associated with their implementation and operation:
Data Quality and Bias :
AI models rely on the training input data for learning patterns and making predictions. If the input data provided for AI model training is biased, incomplete or unrepresentative of the true population, it is bound to lead in biased predictions and inaccurate results thereby exacerbating existing disparities and discrimination. To maintain data quality is a very big challenge.
Adversarial Attacks :
Fraudsters usually attempt manipulating or deceiving AI models by exploiting these vulnerabilities and also by generating adversarial examples which are subtly modified inputs and are designed to evade detection. Adversarial attacks tend to undermine the otherwise effectiveness of AI-based fraud detection systems leading to increased fraud rates, to combat these attacks is a very complex challenge.
Concept Drift :
Concept drift is the phenomenon when the relationships between features and their outcomes change leading to increased false positives and false negatives. The evolving fraud patterns and tactics are responsible to concept drift. All AI models tend to become less effective at detecting new fraud patterns when they are not timely and continuously updated and are not retrained with fresh data, procurement of which is a big challenge.
Over-reliance on Automation :
It stands true that automation does improve efficiency and scalability yet over-reliance on AI-based fraud detection systems without human oversight and intervention can lead to complacency and also missed detections. Human analysts always play a crucial role in interpreting results, investigating alerts and also in making informed decisions that are based on context and domain knowledge.
Regulatory Compliance :
AI-based fraud detection systems have to be compliant with various regulatory requirements and privacy laws like the GDPR or CCPA which impose mandatory restrictions on data usage, model transparency and also on user consent. To maintain compliance is a complex challenge and the failure to comply often results in legal and financial penalties as well as reputational damage for the organization concerned.
Model Interpretability :
AI models with complex deep learning neural networks currently lack interpretability which makes it very challenging for users to understand the reasoning behind their decisions. This lack of model interpretability undermines the trust and accountability in AI-based fraud detection systems when deployed in highly regulated industries.
Cybersecurity Risks :
All AI models and the data which is used for their training and inference are quite vulnerable to cybersecurity threats like data breaches, model poisoning attacks and even the model inversion attacks. Protecting the AI systems against cybersecurity risks is not only crucial for safeguarding the sensitive information and maintaining the integrity of fraud detection processes but also a very complex challenge.
Organizations have to very carefully consider and work towards mitigating these risks when deploying AI-based fraud detection systems for ensuring their effectiveness, reliability and complete compliance with all the mandatory regulatory requirements. Collaboration between data scientists, domain experts and cybersecurity professionals like AI development companies and legal advisors is essential for addressing these challenges and building robust and trustworthy fraud detection capabilities.
The suggested solutions
Addressing the challenges associated with AI-based fraud detection and prevention systems requires a multi-faceted approach which involves technical, organizational and regulatory measures.
Let’s see some suggested potential solutions:
Data Quality Assurance :
A suggested solution is to ensure that all the training data that is used to train AI models be representative, diverse and free from bias. Implementing data pre-processing techniques like data cleaning, normalization and augmentation generally improves the data quality while mitigating biases. Also suggested is to regularly monitor and audit data pipelines for identifying and addressing any data quality issues.
Testing Robustness :
Regularly conducting robustness tests for evaluating the AI models' resilience for adversarial attacks, concept drift and other sources of model degradation is suggested. Also suggested is the use of techniques like adversarial training, model ensembling and robust optimization for enhancing the model’s robustness and reliability.
Human intervention :
it is always advisable to incorporate human oversight and intervention into every AI-based fraud detection and prevention systems to complement these automated processes and mitigate any the challenges of false positives and false negatives. Appropriately empowering all human analysts with tools and interfaces for interpreting the AI model outputs, investigating alerts and providing feedback is essential in improving AI model performance.
Interpretability and Explainability :
The suggested solution for this challenge is to develop interpretable and explainable AI models which easily provide insights into model decisions and reasoning. Using techniques like feature importance analysis, model explanation methods and decision tree visualization are to be implemented for enhancing model interpretability and transparency.
Regulatory Compliance :
It should be ensured that all AI-based fraud detection systems comply with all the applicable mandatory regulatory requirements and privacy laws like GDPR, CCPA and financial regulations (e.g., PCI DSS). Additionally implementing privacy-preserving techniques like data anonymization, differential privacy and federated learning are required for securing sensitive information and maintaining regulatory compliance.
Cybersecurity Measures :
Implementing robust cybersecurity measures to safeguard AI systems against cyber risks using encryption, access controls, secure data transmission protocols and regular security audits is the most important solution to protect all AI models and data from cyber threats like data breaches, model poisoning attacks and adversarial manipulations.
Continuous Monitoring and Evaluation :
It is most effective to continuously be monitoring and evaluating the performance of AI-based fraud detection systems over time. Also using metrics like detection accuracy, false positive rate, false negative rate and model drift for assessing model effectiveness and identifying areas for improvement is essential. Regular update and retraining models with fresh data are advised to adapt to the evolving fraud patterns and also for maintaining performance.
By adopting these suggested solutions, organizations can definitely mitigate the risks and challenges associated with AI-based fraud detection systems and implement robust, reliable and compliant fraud detection capabilities which could effectively safeguard against fraudulent activities while maintaining trust and transparency.
Based on the present trends the predicted forecast for AI technologies in fraud detection and prevention
Based on the present trajectory of AI-based fraud detection trends, let’s see the potential directions of development in the coming future:
Increased Adoption:
The increasing adoption of AI-based fraud detection systems is expected to continue growing across various industries as more and more organizations are seeking effective ways to combat the growing sophisticated fraud schemes.
Advancements in AI Technology :
The continuing advancements in AI technology especially in the domains of machine learning, deep learning and natural language processing will be enabling more accurate, efficient and scalable fraud detection solutions.
Interdisciplinary Collaboration :
Healthy collaboration between data scientists, domain experts, cybersecurity professionals and regulatory experts will become very important in addressing the complex challenges that are associated with AI-based fraud detection like data quality, model interpretability and regulatory compliance.
Enhanced Robustness and Security :
The future will see a greater focus on improving the robustness and security of AI-based fraud detection systems for mitigating the challenging risks such as adversarial attacks, concept drift and also the cybersecurity threats.
Privacy-Preserving Techniques :
The growing adoption of privacy-preserving techniques like the federated learning, differential privacy and the homomorphic encryption will soon be enabling organizations in protecting sensitive data while still implementing AI for fraud detection and prevention.
Privacy-Preserving Techniques :
The growing adoption of privacy-preserving techniques like the federated learning, differential privacy and the homomorphic encryption will soon be enabling organizations in protecting sensitive data while still implementing AI for fraud detection and prevention.
Explainable AI :
The near future will experience an increased demand for explainable AI models that provide insights into model decisions and reasoning especially in regulated industries where transparency and accountability are paramount.
Real-time Detection and Responses:
AI-based fraud detection and prevention systems will be continuing to evolve in real-time detection and their response capabilities thereby enabling organizations in quickly identifying and mitigating fraudulent activities just as they occur.
The future of AI-based fraud detection and prevention looks promising as the ongoing innovation and collaboration drives improvements in effectiveness, efficiency and trustworthiness across various industries. It's pertinent to remain absolutely vigilant and proactive in addressing all emerging challenges and risks for ensuring the continuing success and reliability of AI-based fraud detection solutions.
The role of AI development companies in implementation of ai technology and mitigating the challenges.
AI development companies play a significant role in both the implementation of AI-based fraud detection systems and also in mitigating the challenges associated with them.
Let’s see how:
Expertise in AI Technology :
AI development companies are the experts in AI technology which includes machine learning, deep learning, natural language processing NLP and data analytics. They are capable of implementing this expertise into designs, development and of course the sophisticated fraud detection algorithms and models which are customized to the specific needs and challenges of their clients.
Customization and Integration :
AI development companies have the capabilities of customizing and integrating AI-based fraud detection solutions into the client’s existing systems and workflows thereby ensuring seamless integration and interoperability with other software and technologies that are being used by their clients.
Data Management and Quality Assurance :
AI development companies are capable of efficiently assisting organizations in managing and pre-processing data to ensure its quality, relevance and representativeness for training all the AI models. They can also effectively implement data governance frameworks and best practices to maintain the all important data privacy, security and compliance with all mandatory regulatory requirements.
Model Evaluation and Optimization :
AI development companies provide services to organizations for evaluating the performance of their AI models, identifying areas for improvement and optimizing model parameters and hyper parameters for enhancing detection accuracy, reducing false positives and thereby improving overall efficiency.
Continuous Monitoring and Maintenance :
AI development companies provide through their services provide to their client organizations ongoing support, monitoring and maintenance services to ensure the continued effectiveness and reliability of AI-based fraud detection systems. Their expert team of developers are also capable of assisting organizations in detecting and responding to any emerging fraud threats and also in the evolving regulatory requirements.
Training and Education :
AI development companies through their services also offer training and education programs to help employees of their client organizations understand the capabilities and limitations of AI-based fraud detection as well as all the best practices for implementation, deployment and effective usage. They also through their services guide on all the ethical and responsible AI practices for promoting fairness, transparency and accountability in fraud detection processes.
AI development companies play a very significant role in guiding organizations through the effective implementation of AI-based fraud detection systems and they also assist in mitigating all the challenges associated with them thus successfully safeguard the interests of their clients by helping to protect the client organization against fraud.
AI fraud detection and prevention has revolutionized security across various industries across the world offering advanced capabilities in analyzing vast datasets, detecting anomalies and also by predicting potential risks. From banking and financial services to ai in preventive healthcare, AI-powered solutions are proving to be invaluable in safeguarding the citizens and organizations against fraudulent activities.
With AI continuing to evolve, its role in fraud detection and prevention will gain prominence while driving innovation and enhancing security measures across diverse sectors.
Also with AI detection at the forefront of preventive healthcare and medicine we can be assured that the potential for early intervention and improved patient outcomes is bound to be immense. This indeed is signalling a promising future for AI and promoting overall health and well-being to all!
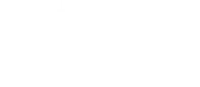
Australia
470 St Kilda Rd
Melbourne Vic 3004
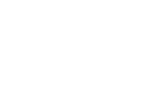
USA
Venture X, 2451 W Grapevine Mills Cir,
Grapevine, TX 76051, United States
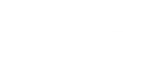
Netherlands
Landfort 64. Lelystad 8219AL
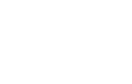
Canada
4025 River Mill Way, Mississauga, ON L4W 4C1, Canada
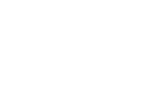
India
4A, Maple High Street, Hoshangabad Road, Bhopal, MP.